Project partner
Background
Classical control concepts are only applicable to a limited extent when handling soft materials. Due to the nonlinear material behavior, there are an infinite number of degrees of freedom, which make automated handling with robots difficult. The simulation of such systems requires complex, computationally expensive models.
As part of the International Research and Training Group Soft Tissue Robotics, possibilities for localization and simulation of soft materials are being investigated at ISW in cooperation with other institutes at the University of Stuttgart and the University of Auckland. In addition, methods are being examined at ISW to directly map input signals to control signals using machine learning on the digital twin. Both of these approaches have been limited to handling soft materials so far and have not looked at motion in a soft environment. Soft environments are present in surgery, for example, where robots are also increasingly used.
Solution approach
A subset of machine learning, reinforcement learning, describes an approach in which an agent learns through trial-and-error. The agent can act within an action space and receives rewards through interaction with its environment, which it tries to maximize. This is possible with a model-free approach, so that learning can occur through interaction with an environment. For industrial or medical applications, learning should not be done on the real system because errors are bound to occur during the learning process, which can be dangerous and costly. As part of the digitalization of engineering, simulations are increasingly being used, which can also be utilized for this purpose. For example, hardware-in-the-loop simulations are used for virtual commissioning, in which the control system is tested using a virtual machine or plant. At ISW, research is being conducted into how these simulation models can be used as a learning environment. This shall be transferred to the interaction with a soft environment.
Expected Results
A simulation environment with soft materials is to be further developed in which a robot can navigate and perform a task using machine learning. In this context, an interaction of materials with different stiffness shall be considered. Based on this simulation environment, the control of robots shall be learned by defining target and boundary conditions enabling a precise movement of a robot in a soft environment. The motion is automatically optimized through experience gained in the course of several simulation runs.
An application area where soft tissue and control technology come together is robotics in surgery. Therefore, a surgical robot that learns how to perform parts of operations automatically will be considered as a use case. In doing so, it must move in soft tissue and interact with it. For example, a stent can be inserted during a catheter operation. In this case, the catheter often has to be moved from the groin area via the blood vessels to the destination.
Get in touch
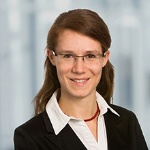
Annika Kienzlen
M.Sc.Research Assistant "Virtual Methods for Production Engineering"