Background
The programming of robots and CNC machines is already supported today by simulation environments. The simulation behaves like the real components in terms of interfaces, parameters and operating modes. By that, realistic test and commissioning situations can be validated through the simulation. Virtual commissioning can achieve a time advantage from the start of development to the operation of a machine and plant by pulling large parts of the control programming to earlier stages. However, the time required for the control development itself is not reduced.
Problem statement
Reinforcement learning has achieved impressive results in many, mostly still non-commercial, areas. A crucial factor is the learning environment within which the agent can interact. For industrial applications (machines, plants and robots), the real system is not possible due to the errors, that the agent inevitably makes for successful learning. These errors, for example collisions, are costly and sometimes dangerous for the system. Instead, artificial environments must be created. However, in the course of virtualization of engineering, such an artificial learning environment is possible without much additional effort. Hardware-in-the-loop (HiL) simulations are state of the art when it comes to virtual commissioning. Here, the real control system is developed, validated and tested in conjunction with a virtual machine or plant. In this project, the approach is to use exactly these HiL simulation models as a learning environment for a cognitive control.
Goals
The goal of the project is to develop a new type of automated control programming using machine learning. The idea is to use the HiL simulation environment for automatic control development and optimization for the first time. This will shorten the software development process and significantly reduce the investment costs for switching to a product variant.
Get in touch
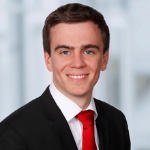
Florian Jaensch
M.Sc.Research Assistant "Virtual Methods for Production Engineering"